AI in Manufacturing: AI Visual Quality Control
Author: Janne Flinck
Introduction
Inspired by the Smart Industry event, we decided to start a series of blog posts that tackle some of the issues in manufacturing with AI. In this first section, we will talk about automating quality control with vision AI.
Manufacturing companies, as well as companies in other industries like logistics, prioritize the effectiveness and efficiency of their quality control processes. In recent years, computer vision-based automation has emerged as a highly efficient solution for reducing quality costs and defect rates.
The American Society of Quality estimates that most manufacturers spend the equivalent of 15% to 20% of revenues on “true quality-related costs.” Some organizations go as high as 40% cost-of-quality in their operations. Cost centers that affect quality in manufacturing come in three different areas:
- Appraisal costs: Verification of material and processes, quality audits of the entire system, supplier ratings
- Internal failure costs: Waste of resources or errors from poor planning or organization, correction of errors on finished products, failure of analysis regarding internal procedures
- External failure costs: Repairs and servicing of delivered products, warranty claims, complaints, returns
Artificial intelligence is helping manufacturers improve in all these areas, which is why leading enterprises have been embracing it. According to a 2021 survey of more than 1,000 manufacturing executives across seven countries interviewed by Google Cloud, 39% of manufacturers are using AI for quality inspection, while 35% are using it for quality checks on the production line itself.
Top 5 areas where AI is currently deployed in day-to-day operations:
- Quality inspection 39%
- Supply chain management 36%
- Risk management 36%
- Product and/or production line quality checks 35%
- Inventory management 34%
Source: Google Cloud Manufacturing Report
With the assistance of vision AI, production line workers are able to reduce the amount of time spent on repetitive product inspections, allowing them to shift their attention towards more intricate tasks, such as conducting root cause analysis.
Modern computer vision models and frameworks offer versatility and cost-effectiveness, with specialized cloud-native services for model training and edge deployment further reducing implementation complexities.
Solution overview
In this blog post, we focus on the challenge of defect detection on assembly and sorting lines. The real-time visual quality control solution, implemented using Google Clouds Vertex AI and AutoML services, can track multiple objects and evaluate the probability of defects or damages.
The first stage involves preparing the video stream by splitting the stream into frames for analysis. The next stage utilizes a model to identify bounding boxes around objects.
Once the object is identified, the defect detection system processes the frame by cutting out the object using the bounding box, resizing it, and sending it to a defect detection model for classification. The output is a frame where the object is detected with bounding boxes and classified as either a defect or not a defect. The quick processing time enables real-time monitoring using the model’s output, automating the defect detection process and enhancing overall efficiency.
The core solution architecture on Google Cloud is as follows:
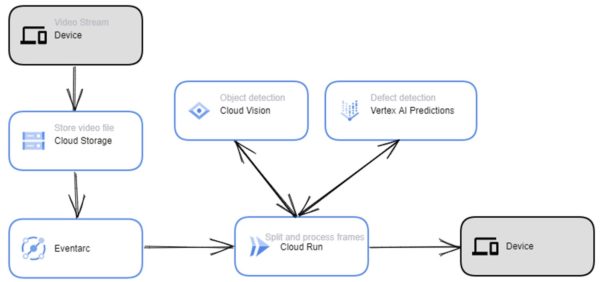
Implementation details
In this section I will touch upon some of the parts of the system, mainly what it takes to get started and what things to consider. The dataset is self created from objects I found at home, but this very same approach and algorithm can be used on any objects as long as the video quality is good.
Here is an example frame from the video, where we can see one defective object and three non-defective objects:
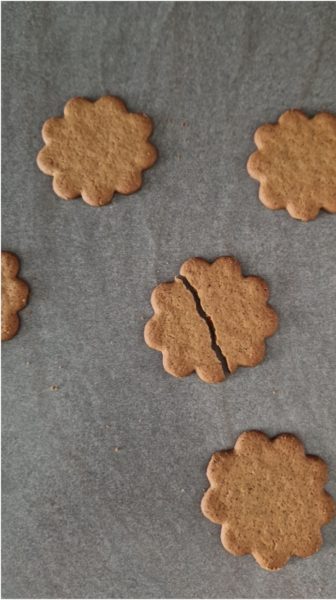
We can also see that one of the objects is leaving the frame on the right side and another one is entering the frame from the left.
The video can be found here.
Datasets and models overview
In our experiment, we used a video that simulates a conveyor belt scenario. The video showed objects moving from the left side of the screen to the right, some of which were defective or damaged. Our training dataset consists of approximately 20 different objects, with four of them being defective.
For visual quality control, we need to utilize an object detection model and an image classification model. There are three options to build the object detection model:
- Train a model powered by Google Vertex AI AutoML
- Use the prebuilt Google Cloud Vision API
- Train a custom model
For this prototype we decided to opt for both options 1 and 2. To train a Vertex AI AutoML model, we need an annotated dataset with bounding box coordinates. Due to the relatively small size of our dataset, we chose to use Google Clouds data annotation tool. However, for larger datasets, we recommend using Vertex AI data labeling jobs.
For this task, we manually drew bounding boxes for each object in the frames and annotated the objects. In total, we used 50 frames for training our object detection model, which is a very modest amount.
Machine learning models usually require a larger number of samples for training. However, for the purpose of this blog post, the quantity of samples was sufficient to evaluate the suitability of the cloud service for defect detection. In general, the more labeled data you can bring to the training process, the better your model will be. Another obvious critical requirement for the dataset is to have representative examples of both defects and regular instances.
The subsequent stages in creating the AutoML object detection and AutoML defect detection datasets involved partitioning the data into training, validation, and test subsets. By default, Vertex AI automatically distributes 80% of the images for training, 10% for validation, and 10% for testing. We used manual splitting to avoid data leakage. Specifically, we avoid having sets of sequential frames.
The process for creating the AutoML dataset and model is as follows:
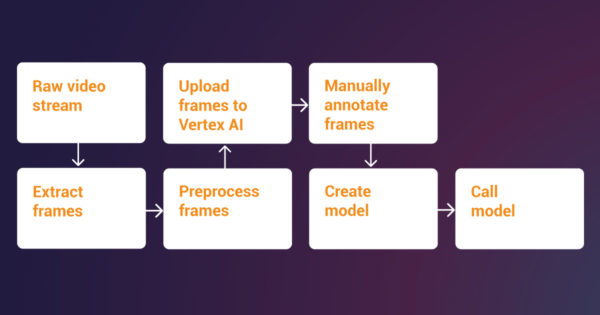
As for using the out-of-the-box Google Cloud Vision API for object detection, there is no dataset annotation requirement. One just uses the client libraries to call the API and process the response, which consists of normalized bounding boxes and object names. From these object names we then filter for the ones that we are looking for. The process for Vision API is as follows:
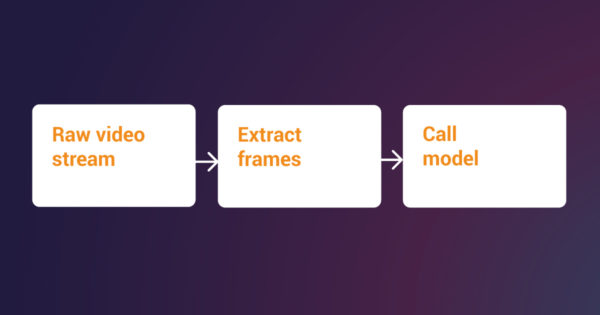
Why would one train a custom model if using Google Cloud Vision API is this simple? For starters, the Vision API will detect generic objects, so if there is something very specific, it might not be in the labels list. Unfortunately, it looks like the complete list of labels detected by Google Cloud Vision API is not publicly available. One should try the Google Cloud Vision API and see if it is able to detect the objects of interest.
According to Vertex AI’s documentation, AutoML models perform optimally when the label with the lowest number of examples has at least 10% of the examples as the label with the highest number of examples. In a production case, it is important to capture roughly similar amounts of training examples for each category.
Even if you have an abundance of data for one label, it is best to have an equal distribution for each label. As our primary aim was to construct a prototype using a limited dataset, rather than enhancing model accuracy, we did not tackle the problem of imbalanced classes.
Object tracking
We developed an object tracking algorithm, based on the OpenCV library, to address the specific challenges of our video scenario. The specific trackers we tested were CSRT, KCF and MOSSE. The following rules of thumb apply in our scenario as well:
- Use CSRT when you need higher object tracking accuracy and can tolerate slower FPS throughput
- Use KCF when you need faster FPS throughput but can handle slightly lower object tracking accuracy
- Use MOSSE when you need pure speed
For object tracking we need to take into account the following characteristics of the video:
- Each frame may contain one or multiple objects, or none at all
- New objects may appear during the video and old objects disappear
- Objects may only be partially visible when they enter or exit the frame
- There may be overlapping bounding boxes for the same object
- The same object will be in the video for multiple successive frames
To speed up the entire process, we only send each fully visible object to the defect detection model twice. We then average the probability output of the model and assign the label to that object permanently. This way we can save both computation time and money by not calling the model endpoint needlessly for the same object multiple times throughout the video.
Conclusion
Here is the result output video stream and an extracted frame from the quality control process. Blue means that the object has been detected but has not yet been classified because the object is not fully visible in the frame. Green means no defect detected and red is a defect:
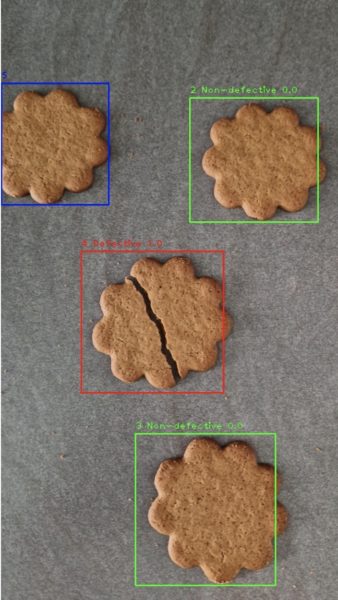
The video can be found here.
These findings demonstrate that it is possible to develop an automated visual quality control pipeline with a minimal number of samples. In a real-world scenario, we would have access to much longer video streams and the ability to iteratively expand the dataset to enhance the model until it meets the desired quality standards.
Despite these limitations, thanks to Vertex AI, we were able to achieve reasonable quality in just the first training run, which took only a few hours, even with a small dataset. This highlights the efficiency and effectiveness of our approach of utilizing pretrained models and AutoML solutions, as we were able to achieve promising results in a very short time frame.
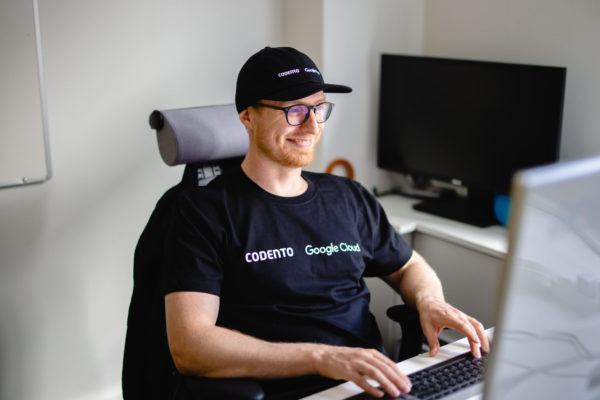
About the author: Janne Flinck is an AI & Data Lead at Codento. Janne joined Codento from Accenture 2022 with extensive experience in Google Cloud Platform, Data Science, and Data Engineering. His interests are in creating and architecting data-intensive applications and tooling. Janne has three professional certifications in Google Cloud and a Master’s Degree in Economics.
Please contact us for more information on how to utilize artificial intelligence in industrial solutions.